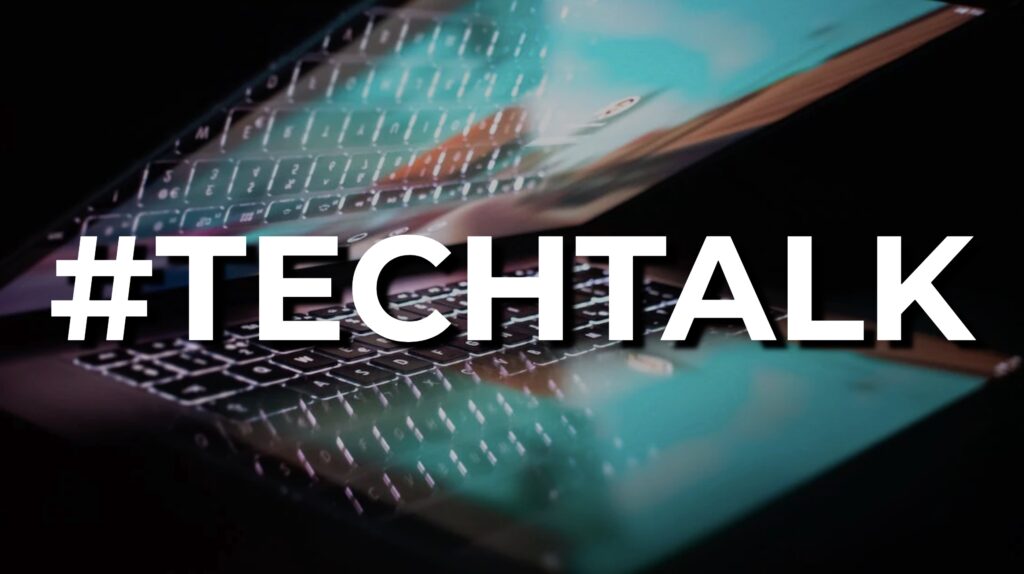
In today’s data-driven landscape, the integration of data fuels unprecedented value. Organisations strive to optimise their data assets, emphasising the principles of Findability, Accessibility, Interoperability, and Reusability (FAIR). These principles are a priority for the UK’s Geospatial Commission, ONS and the wider government via the Data Maturity Assessment framework. They’re also important to collaborations like Fostering Fair Data Practices in Europe and Bill & Melinda Gates Foundation. They form the bedrock for a new era in data utilisation.
The first step in this journey is finding data, a process where the ‘Findable‘ and ‘Reusable’ in FAIR becomes pivotal. While standards play a crucial role, the need for well-crafted data descriptions is often overlooked. Many data catalogues house poorly described datasets, creating challenges for users in discerning relevance.
In conversations with data teams and governance stakeholders, it’s clear that those responsible for metadata often lack sufficient time, resources, or guidance. Review processes are limited, leaving room for improvement. As we explore available guidance, it’s evident that the focus varies across organisations, but there is often little emphasis on readability and accessibility of language.
This post delves into valuable guidance from organisations like the Environmental Information Data Centre, Data.gov.uk, and the NOAA. However, the scarcity of comprehensive guidance highlights an opportunity for better practices in dataset descriptions.
While there’s a dearth of guidance, there are tools to enhance language and readability. Resource examples such a plain language guide, the Gov.uk style guide, and readability apps such as Hemingway Editor offer starting points. Additionally, readability tools like Readable.com provide insights into sentence structure and language features.
Looking ahead, the role of AI tools like ChatGPT in restructuring and redrafting descriptions is on the horizon. While caution is advised, these tools could become valuable aids in the data description process.
Authoring guidance in the wild
I thought it would be useful to highlight some of the guidance that I have found, out there in the wild:
Environmental Information Data Centre (EIDC)
The Environmental Information Data Centre (EIDC) is part of the Natural Environment Research Council’s (NERC) Environmental Data Service and is hosted by the UK Centre for Ecology & Hydrology (UKCEH). They manage nationally-important datasets concerned with the terrestrial and freshwater sciences.
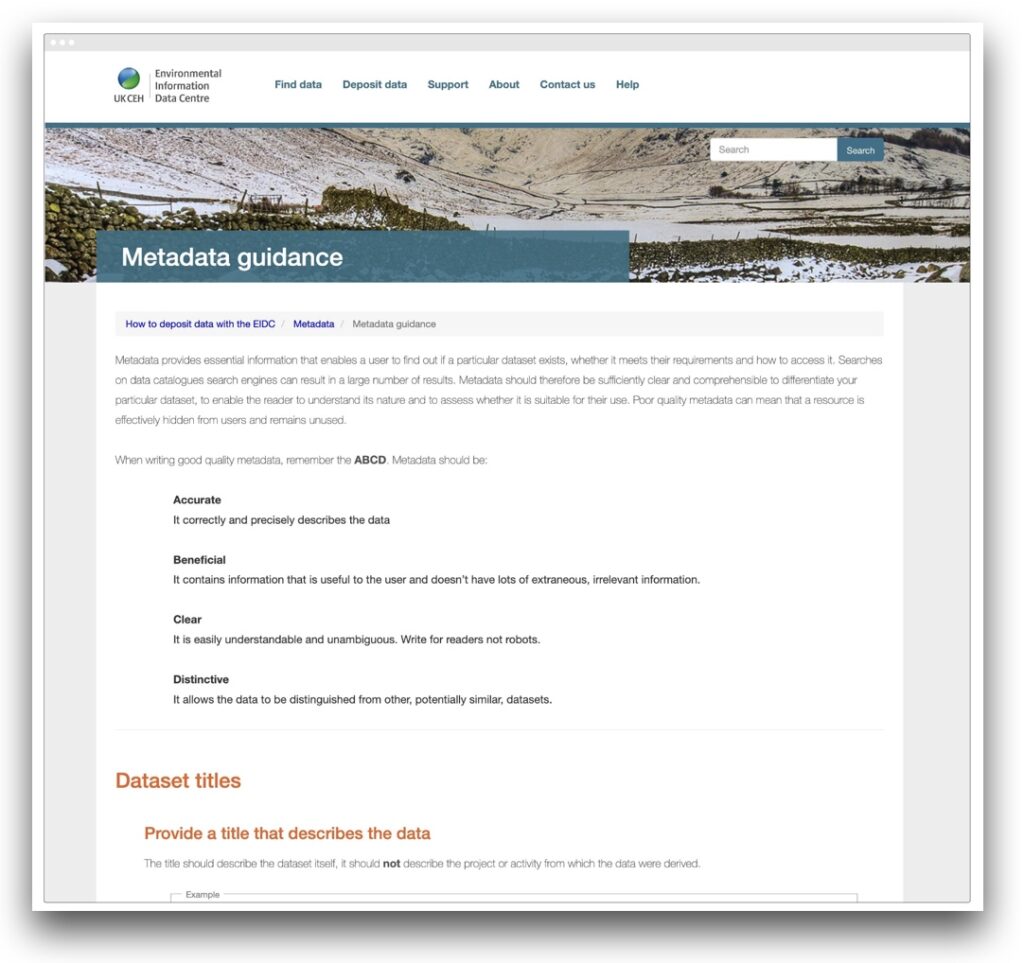
Links: eidc.ac.uk/deposit/metadata and eidc.ac.uk/deposit/metadata/guidance
The EIDC give examples of both how to describe a dataset and how to give it a title.
Data.gov.uk
Central government data cataloguing guidance covering data publishing on data.gov.uk and gov.uk in general.
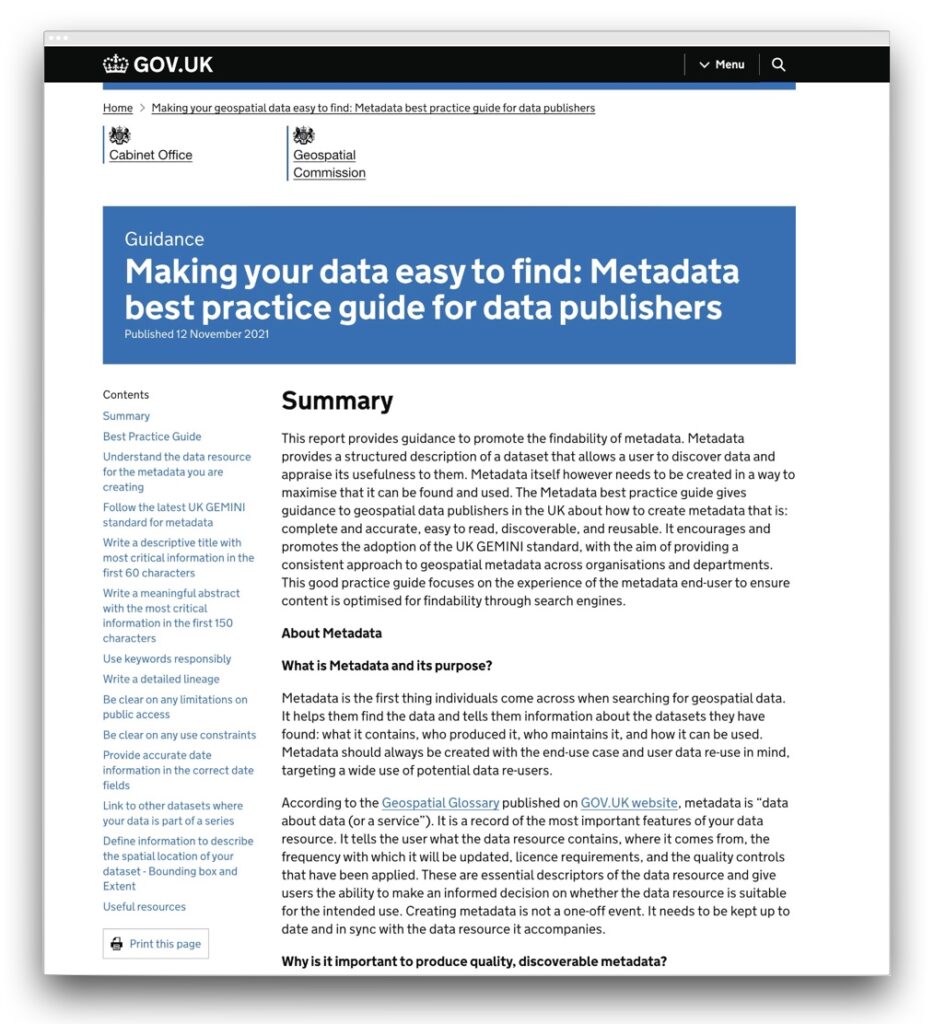
Making your data easy to find: Metadata best practice guide for data publishers covering gov.uk and data.gov.uk
Again this resource gives guidance and examples of both how to describe a dataset and how to give it a title, in terms of what should be covered, so that it is easy to find and understand.
National Oceanographic & Atmospheric Administration (NOAA) / National Centres for Environmental Information (NCEI)
The National Oceanographic and Atmospheric Administration (NOAA) is a US government agency, the National Centres for Environmental Information (NCEI) provides environmental data, products, and services covering the depths of the ocean to the surface of the sun to drive resilience, prosperity, and equity for current and future generations.
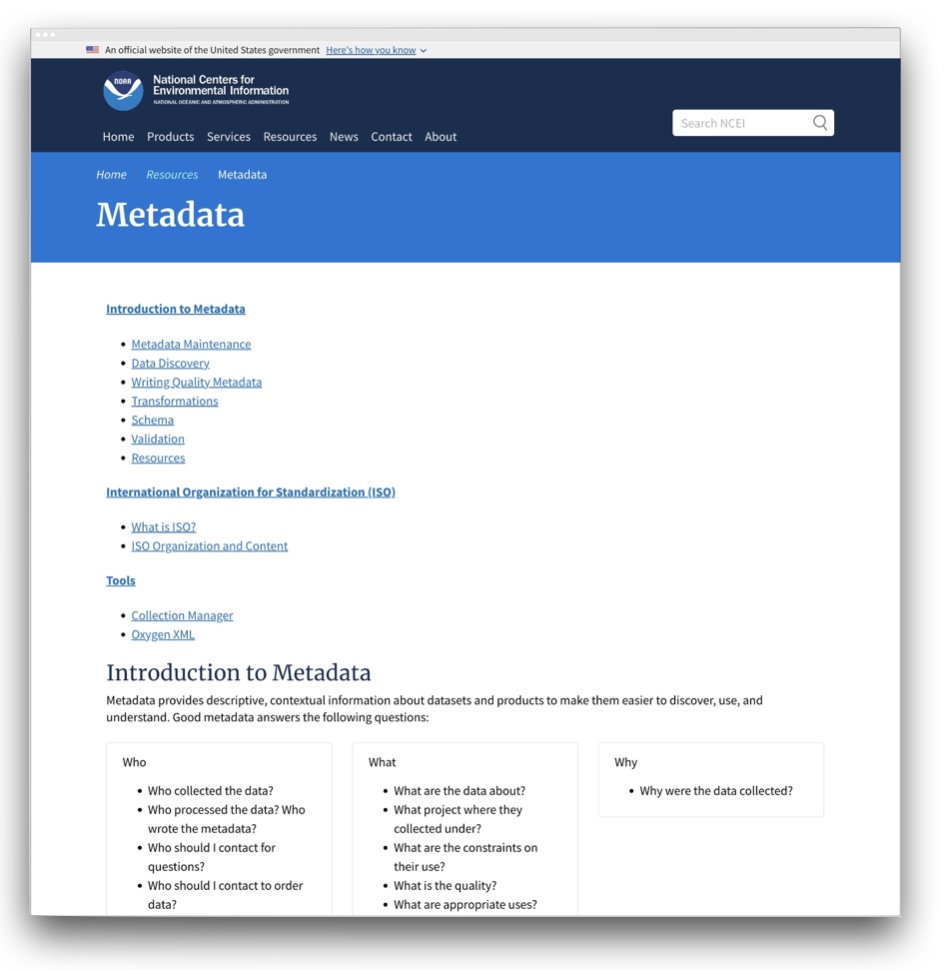
Links: www.ncei.noaa.gov/resources/metadata and www.ncei.noaa.gov/resources/metadata#write
This guidance focuses on the need for plain language and the use of a template / style guide.
Guidance Summary
The guidance out there tends to focus on the particular priorities of the publishing organisation, and the aspects that are most important to them. It is notable that there is currently less recognition of the readability and accessibility of the language used in these guides for publishers, which is unfortunately reflected in the quality of some of the language used in published dataset descriptions.
If there is guidance out there that I’ve missed, that you’d recommend, then I’d love to hear about it.
Some tools that can help
There are useful tools and resources that can be used to help check the language and readability of descriptive text. These could be used to test for readability, and be a useful step in improving the text that we see describing datasets in lots of catalogues. Suchtools could help to improve accessibility and consistency of style across multiple dataset descriptions.
Style Guidance
As a starting point, here are some relevant and useful style and language guides:
- a plain language guide by Eval Academy
- the Gov.uk style guide – Content design: planning, writing and managing content. How to write well for your audience, including specialists
- note the section: “How people read” and recommendations for choosing titles and writing short summaries
- the British Dyslexia Association (BDA)’s dyslexia friendly style guide
Readability apps
There are also quite a wide range of readability tools such as:
Hemingway Editor: an online app which brands itself as “like a spellchecker, but for style”. The Hemingway Editor helps you make your text clear, by identifying all the disorganised and excessive prose in the copy. Wordy, long sentences are highlighted for being too complicated. As well as adverbs, uses of the passive voice and phrases with simpler alternatives. All factors contribute to reading ease. By making the small adjustments recommended, you can see the readability score improve.
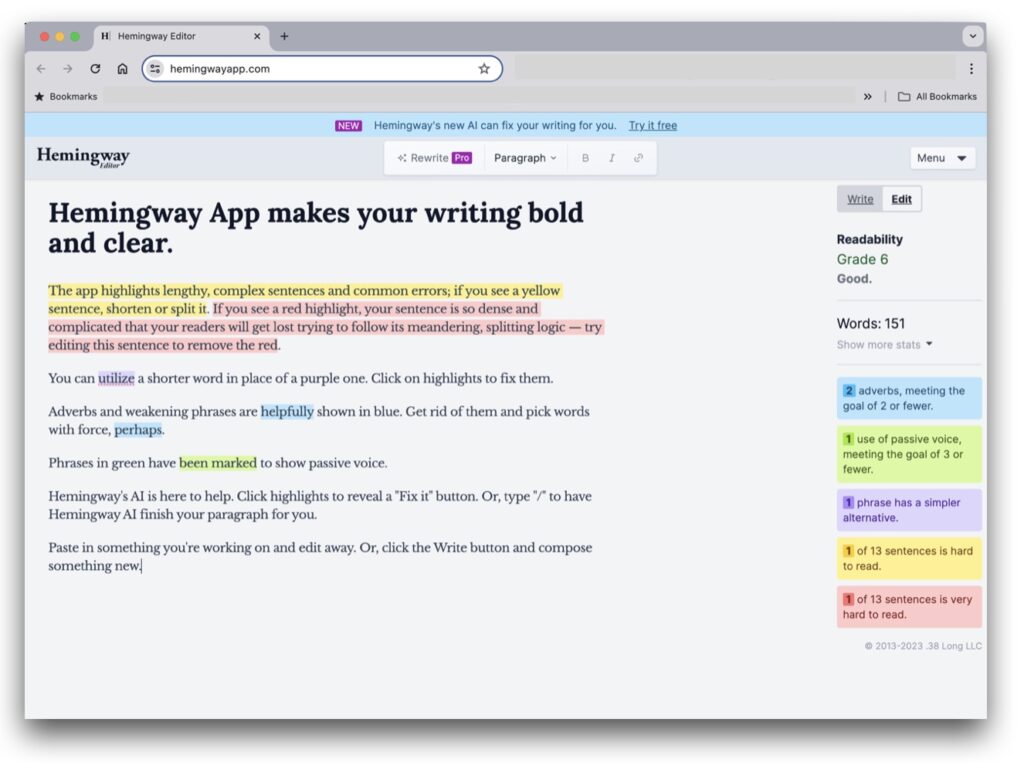
Readability analyzer: this tool not only analyses readability, but also gives you different ways to explore your text, including paragraph-by-paragraph level readability assessments and extra tools such as Difficult and Extraneous Word Finder, Passive Voice Detector and more.
Readable.com: with a whole host of ways to make your text readable, they have a free online version of their tool that gives you access for a limited time. This is made for occasional use but you can identify the difficult sentences, long words, and other detrimental features of your writing. They also have a good explainer of the different readability scores and grades used across many of these different services.
Other useful tools
A number of the above tools will highlight profanities, jargon or buzzwords. These examples below can do this separately:
- Readable’s Buzzword Detector
- Readable’s Profanity Detector
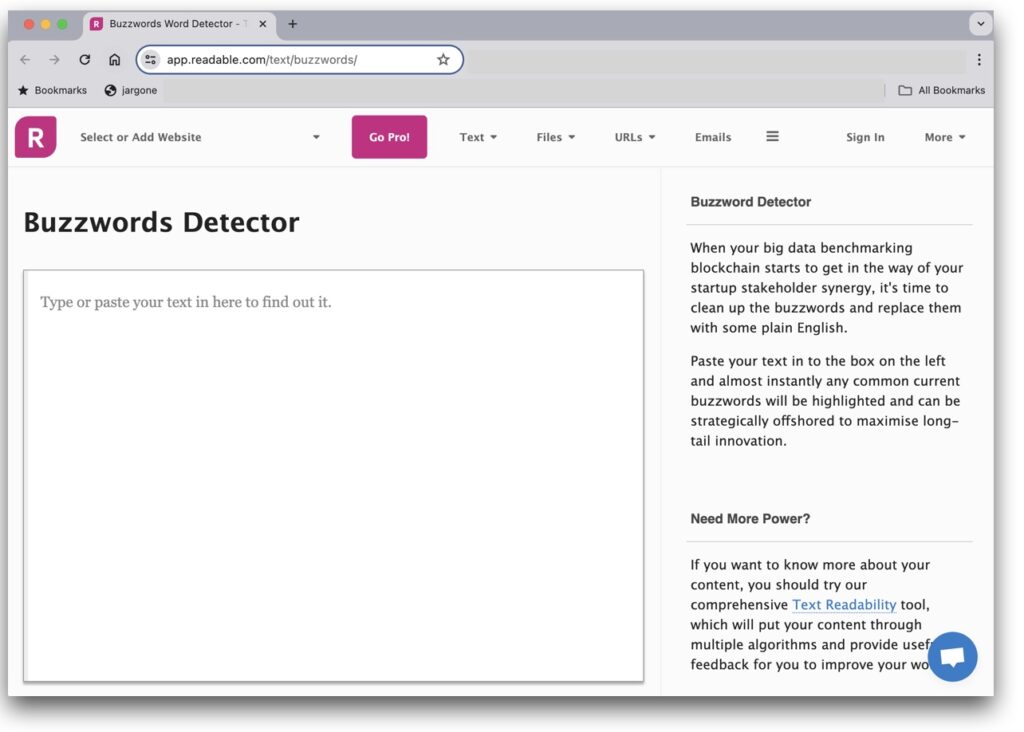
Generative AI
I suspect that over time we will be able to use AI tools like ChatGPT for analysing and helping to restructure and redraft descriptions. These should be used with care, and you should always review text produced before using it, but they could become a useful aid to the process.
Some prompts to consider:
"I've crafted this complex paragraph: '[Your paragraph here]'. Can you provide feedback on its structure and clarity?"
“make this dataset title more persuasive: '[Your dataset title here]'
“write a meta description for [webpage]” or “create a compelling meta description for [your example text]”
“write a title tag for [webpage or descriptive text]” or “create an engaging title tag for [webpage or descriptive text]”
This Prompt writing guidance is a good starting point, but in summary:
- Keep the prompt open-ended to allow for a range of responses.
- Use clear and concise language to avoid confusion.
- Encourage the writer to engage their imagination and emotions.
- Use specific details and sensory language to make the prompt feel real and important.
- Tailor the prompt to the specific genre and purpose of the writing.
- Consider the intended audience and objectives of the writing.
If you are embarking on a journey toward clearer, more impactful data descriptions or have experience in this area. Share your experiences, tools, or guidance that have improved your data cataloguing practices. In championing the cause of FAIR data principles it is important to elevate the accessibility of how we describe data.
Reach out and share your insights.